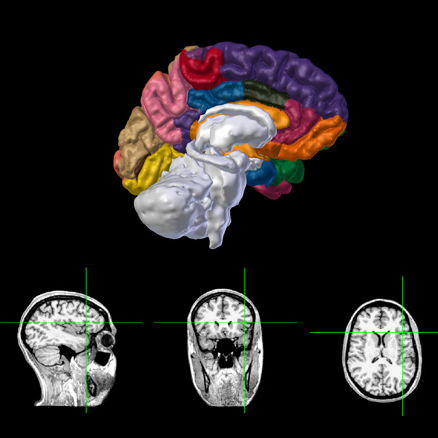
Anatomy
3D surface base visualization of the hemispheres and subcortical regions with MRI/CT slices viewer.
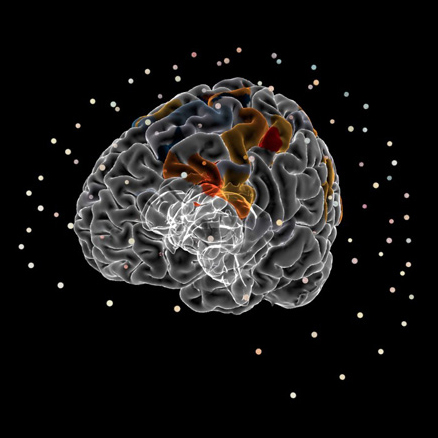
MEG
Interactive visualization of sensors and source space EEG data.
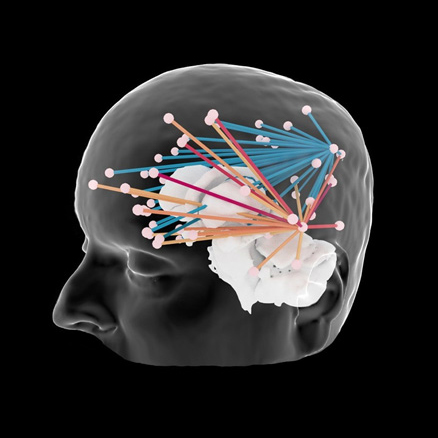
Connectivity
Interactive connectivity between MEG/EEG sensors, cortical labels and invasive electrodes.
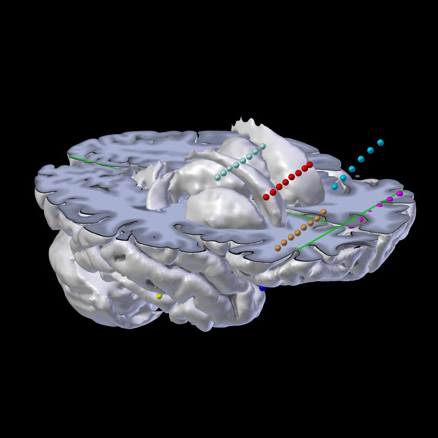
Depth Electrodes
Interactive visualization of Depth Electrodes, ECOG, depth, DBS, etc.
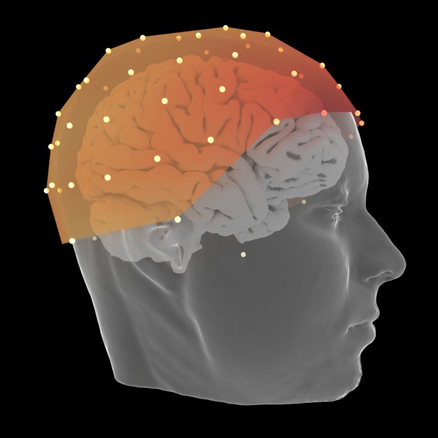
EEG
Interactive visualization of sensors and source space EEG data.
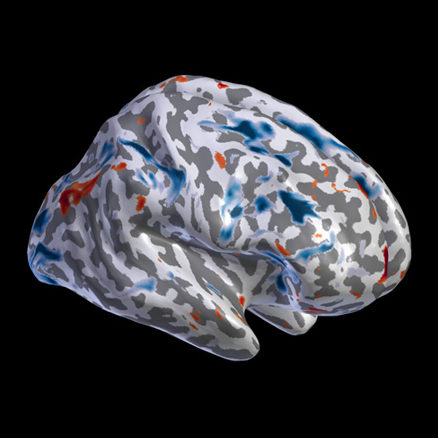
fMRI
Contrast maps and resting state 4D fMRI/PET data projected on the cortical and subcortical surfaces.
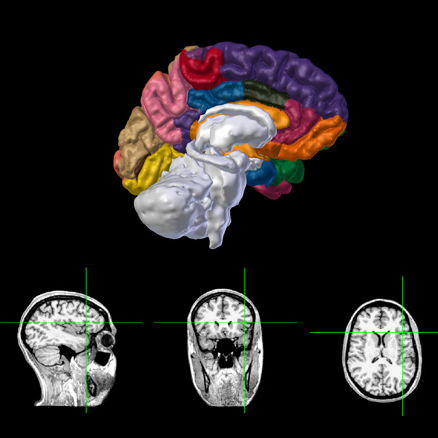
Anatomy
3D surface base visualization of the hemispheres and subcortical regions with MRI/CT slices viewer.
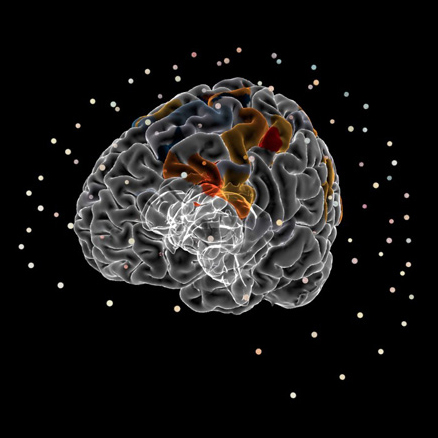
MEG
Interactive visualization of sensors and source space EEG data.
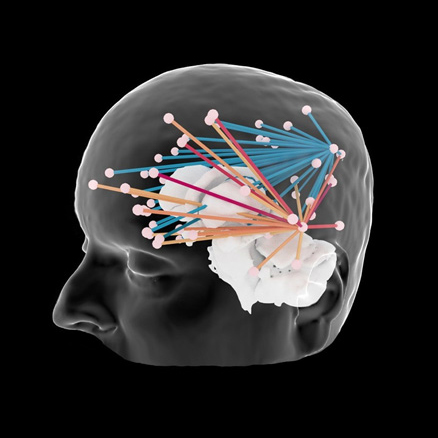
Connectivity
Interactive connectivity between MEG/EEG sensors, cortical labels and invasive electrodes.
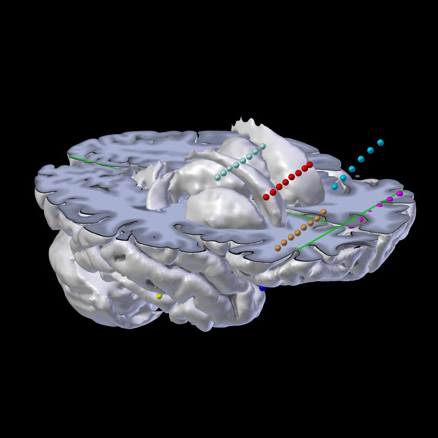
Depth Electrodes
Interactive visualization of Depth Electrodes, ECOG, depth, DBS, etc.
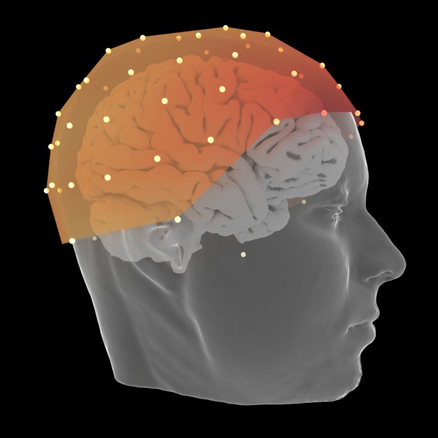
EEG
Interactive visualization of sensors and source space EEG data.
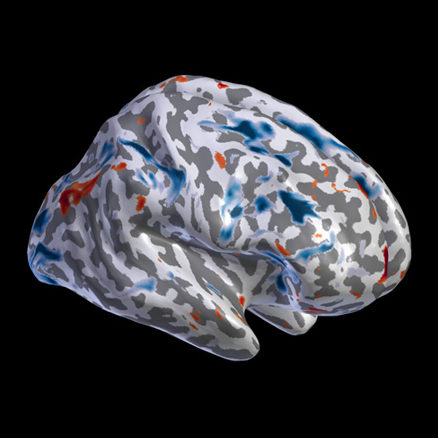
fMRI
Contrast maps and resting state 4D fMRI/PET data projected on the cortical and subcortical surfaces.
Why MMVT
Brain activity in human subjects is often measured with electroencephalography (EEG), magnetoencephalography (MEG), magnetic resonance imaging (fMRI), and/or positron emission tomography (PET). Each technique has limitations and advantages in terms of temporal and spatial resolution. Multimodal neuroimaging allows researchers to overcome these limitations by combining two or more modalities with different limitations. This approach allows researchers to synthesize knowledge, improving our understanding of the structure and function of the brain by utilizing complementary physical and physiological sensitivities. There is an increasing demand for this type of study and the tools to support it.
Clinics and researchers already use multiple unimodal methods in one of several ways, producing the multimodal approaches highlighted by these authors. Fusing two unimodal datasets as done with EEG and fMRI in epilepsy clinics is one such approach.
The growth of these multimodal approaches face challenges in both collection and analysis. Initial problems came from gathering sufficient data sets from several unimodal methods. Large-scale databases that store and share multiple data modalities for groups of subjects, such as the Transform Deep Brain Stimulation (Transform DBS) and Human Connectome, projects offer a solution to this issue. Another issue in data collection is the noise introduced during simultaneous recording or imaging processes, for example, the mechanical noise produced by EEG tools in an MR setting. The artifacts pose a unique challenge to data preprocessing in multimodal approaches that can be addressed with several open source tools to optimize this pipeline.
Likewise, post-processing analysis poses challenges to multimodal research as well. First, one needs to decide whether to analyze the different modalities in parallel, sequentially or jointly and symmetrically. While parallel and sequential analysis methods allow researchers to use traditional unimodal analysis, true data fusion to analyze different modalities jointly requires the development
of new analysis methods. The challenges and possible solutions for these analyses have been comprehensively reviewed. A first common step in analyzing data collected by different instruments is the challenge of transforming the coordinate systems of the different modalities to joint space. Different spatial resolutions and references make this a challenge. Our coregistration graphical user interface uses a shared coordinate system based on the subject anatomy.
Lastly, as the analysis of each unimodal becomes increasingly complex due to the growing dimensionality of data, so does the difficulty of visualizing data in a clear and interactive way. This difficulty multiples rapidly as several modalities are analyzed and inspected simultaneously. Limitations in data visualization are critical barriers to hypothesis generation.
The high dimensionality of data and complexity of models used in current analyses have created a need to improve data visualization. In data-driven studies, the ability to flexibly explore data facilitates the generation of secondary hypotheses. These secondary hypotheses can produce richer analyses that make the most out of data that has already been collected. Researchers will be able to spend less time plotting and calculating simple statistics, increasing the efficiency of their data exploration.
Since multimodal data is highly complex and often contains multiple subprocesses, any visualization that represents the data in an incomplete form might lead to biases of the researcher or reader. Researchers will often use the most representative example of a single instance, which can mislead the reader. Multimodal visualization allows for a complete representation by representing more of the information simultaneously. Interactive media in journals published digitally has also helped correct for visualization bias. Our tool has an interactive interface that would allow readers to visualize data from any perspective, reducing this visualization purposes. Thus, this tool is essential for clarity and reduced bias in academic journals and data exploration, especially in multi-modal data sets.
A non-trivial challenge in any visual tool is to allow users to explore data to transform and manipulate the representations in multiple dimensions. The user can view data in sulci, which are visually obstructed gyri, on an inflated brain as well as voxel-based slices using MMVT. Our tool allows exploration not just in more than one modality, but also across frequencies. Visually representing these domains within the view is critical to the development of multimodal approaches.
Another visualization challenge is to visualize the data naturally and intuitively. For example, MMVT allows the user to visualize neural activity in regions of interest, which can be much more informative than voxel-based activity visualizations. Results from many analyses are presented using 2D slices instead of 3D space due to visualization difficulties. This is very intuitive because the data is recorded volumetrically in 3D space. MMVT combines and surpasses all the information that previously has been presented using several slice views by using one intuitive 3D visualization. Similarly, 3D visualization can improve presentations of results in subcortical structures, which are often visualized using slices. Lastly, a significant challenge in visualization is controlling the amount of information presented.
For example, a good visualization needs to provide the user with ways to overcome Occlusions. Occlusions may occur due to using a specific point of view, visualizing subcortical and cortical structures simultaneously and by using a surface type that is not suitable (for example presenting activity in sulci using a pial surface). Another challenge in controlling the amount of information presented is by reducing complexity when possible. For example, enabling exploring electrodes one by one in a structured way or by masking specific regions of interest according to a chosen parameter.
The central purpose of MMVT is to act as a support tool for exploring neuroimaging data. The key functional concept of MMVT is providing common ground between existing specialized analysis tools, not replacing them. MMVT provides a novel approach to visualization and data exploration, but incorporates existing tools for unimodal data processing. In this way, it can be used alongside existing analysis pipelines or integrated into the experimental design.
The advantage of MMVT is that, unlike self-contained analysis packages with visualization capabilities, MMVT does not focus primarily on the analysis of data. Instead, it focuses on creating a means of simultaneous data exploration between neuroimaging modalities. This enables researchers or clinicians to dynamically explore multiple neuroimaging modalities both in the individual or group level. For clinicians, this gives the user a means of visually representing separate clinically relevant tools for diagnostics, prognostics, and so on. For researchers, the advantage is the flexibility that allows for intuitive visualization of complex spatiotemporal structures.
Learn what you can do with the tool
- Visualize multi-modality functional and statistical data on the cortex and subcortical surfaces as well as the activity of invasive electrodes
- Examine highly accurate 3D visualization of neuroanatomy, including the location of invasive electrodes relative to brain structures
- Pre-processing full pipelines, from raw data to statistical maps